ML Security: AI Incident Response Plans and Enterprise Risk Culture
May 10, 2023 • 31 min read
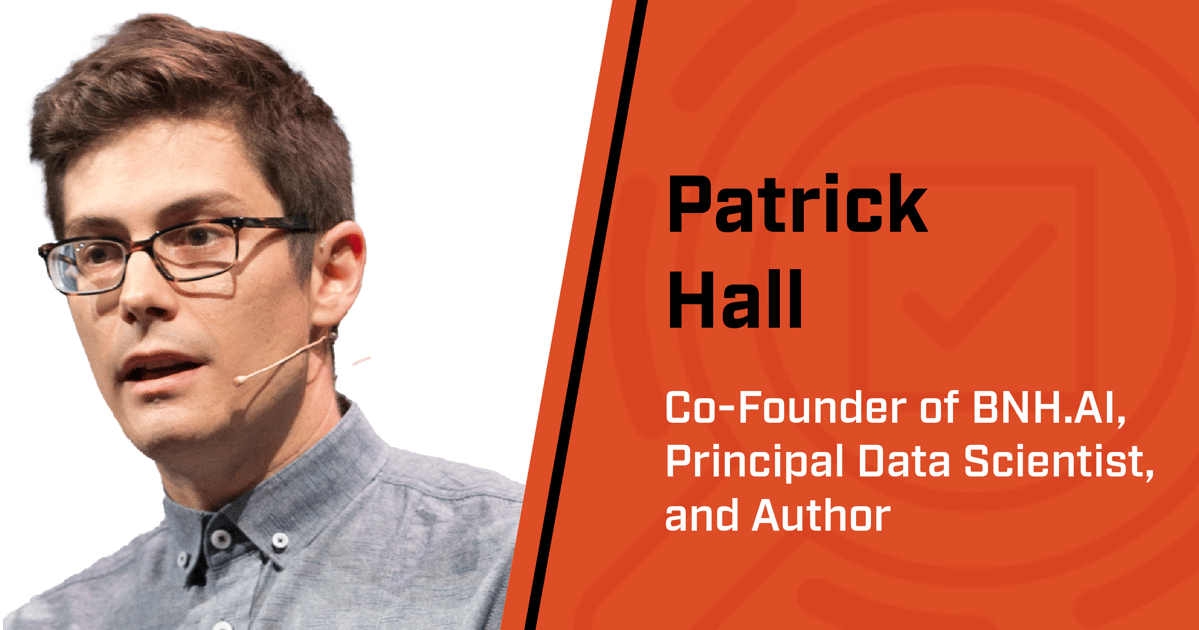
In this episode of The MLSecOps Podcast, Patrick Hall, co-founder of BNH.AI and author of "Machine Learning for High-Risk Applications," discusses the importance of “responsible AI” implementation and risk management. He also shares real-world examples of incidents resulting from the lack of proper AI and machine learning risk management; supporting the need for governance, security, and auditability from an MLSecOps perspective.
YouTube:
Audio Only:
This episode also touches on the culture items and capabilities organizations need to build to have a more responsible AI implementation, the key technical components of AI risk management, and the challenges enterprises face when trying to implement responsible AI practices - including improvements to data science culture that some might suggest lacks authentic “science” and scientific practices.
Also discussed are the unique challenges posed by large language models in terms of data privacy, bias management, and other incidents. Finally, Hall offers practical advice on using the NIST AI Risk Management Framework to improve an organization's AI security posture, and how BNH.AI can help those in risk management, compliance, general counsel and various other positions.
Introduction 0:07
Welcome to The MLSecOps Podcast presented by Protect AI. Your hosts, D Dehghanpisheh, President and Co-Founder of Protect AI, and Charlie McCarthy, MLSecOps Community Leader, explore the world of machine learning security operations, aka, MLSecOps.
From preventing attacks to navigating new AI regulations, we'll dive into the latest developments, strategies, and best practices with industry leaders and AI experts. This is MLSecOps.
D Dehghanpisheh 0:38
Welcome back to The MLSecOps Podcast, everybody. Our guest today is Patrick Hall. Patrick is a principal data scientist and co-founder of BNH.AI. He's also a faculty member at the George Washington University and a co-author of a new book called Machine Learning for High-Risk Applications. Welcome to the show, Patrick.
Patrick Hall 0:58
Hey, great to be here.
Charlie McCarthy 1:00
Yeah, absolutely. Thanks for being here. So, follow up question. BNH.AI, that’s a law firm, right? What is a data scientist doing at a law firm? What’s that about?
Patrick Hall 1:12
That's a great question. And I guess one thing I should say before we get too far into this is I am not a lawyer, and nothing I'm going to say is legal advice. And if you want legal advice, reach out to BNH.AI, where there are real attorneys. So, what is a data scientist doing at a law firm? Well, back around 2016, I was out in Silicon Valley working for a company called H2O.AI, and we started getting into this field of so called “Explainable AI,” which is yet another buzzword that we could talk about.
But really what we wanted to do was fairly straightforward. We wanted to develop ways for large financial institutions to comply with some of their regulatory mandates to explain credit lending decisions. And what I found in that process is I was oftentimes the last data scientist standing in a room full of attorneys. And my co-founder, Andrew Burt at BNH.AI had the same experience from the opposite direction. He was like the last attorney trying to work with the data scientists.
And so, I think in this field of whatever we want to call it, Responsible AI, Trustworthy AI, AI Safety– [who] knows what it's actually called– we're going to run into laws. And I think that myself and Andrew were some of the first people that sort of realized this and took it upon ourselves to try to address a commercial need there. And I think we've shown that there is at least some commercial need there.
Charlie McCarthy 2:36
Great. So, in your time at BNH or even prior, can you think of and maybe share with us one or two real world examples of incidents, and maybe harmful outcomes that resulted from a lack of proper AI and machine learning risk management?
Patrick Hall 2:56
Yes, I can. Being a law firm, we never speak about our sort of client engagements and even who our clients are. But I also sit on the board of the AI incident database which is a great public repository. Very entertaining reading. Anything from entertaining to really sad. But if it's not something you've seen before, I'd urge you to go check out the AI Incident Database.
And one that really comes to mind from that database is - and I actually didn't even hear about this in real time - the entire government, meaning the cabinet, the entire cabinet of a modern parliamentary democracy in the Netherlands was forced to resign in 2021 because their use of an algorithm for fraud detection in government services mistargeted tens of thousands of people and, of course, disproportionately minorities.
And so I always like to point that one out as one of the major AI incidents that I find most people haven't heard of, and I didn't hear of in real time myself. But misuse of algorithms has caused the higher government of a modern democracy to resign.
And we can go in any other direction from there. Autonomous systems injuring people to a kind of funny one where, I believe it was a Chinese actress, was receiving a ton of jaywalking tickets because her face was on a bus and so her face was recognized as jaywalking many times. And so there's thousands of reports of public AI incidents in this database, and they range from the humorous to the really sad and gory and violent.
D Dehghanpisheh 4:40
So, Patrick, one of the things you've talked about in your book and its title is Machine Learning for High-Risk Applications. And it's advertised primarily as providing a holistic framework and describing approaches to responsible AI.
But there are a lot of other terms floating around. Trusted AI, Ethical AI…
How do you define Responsible AI, and is that an appropriate term? Is there a different term that we should be using?
Patrick Hall 5:08
So, much like the field of data science or AI, whatever we even want to call the broader field, right? I'm not even sure we have a good name for that. Just as being an immature science, we have a lot of issues with vocabulary. And Responsible AI, Trusted AI, AI Safety, Ethical AI, whatever it is we choose to call it, it's not well defined. It's overloaded. I don't think that's helpful for anyone. And I think when you see sort of the major players in the tech world laying off their Responsible AI teams, that's a sign that there's at least real commercial problems with that notion of Responsible AI.
I do hear Responsible AI used a lot more in government and civil society circles, where I think it actually makes a lot more sense. It's really hard for a corporation to know and act on what would be possible when it comes to a very complicated technology like AI. And as for the book, I'm sort of at the whims of editors and marketing professionals, and I don't object to it so badly that I asked to have it taken off the book.
And one bit of trivia that I should say… The original title of the book back in 2020 was going to be Responsible AI. And having that bat around in my head for a couple of months, I just ended up feeling like “risk management” is a much more useful framing of whatever it is we're trying to do than Responsible AI. And we can talk about that more if it's interesting to your audience or not.
D Dehghanpisheh 6:47
Yeah, well, one of the things I think that the audience does find interesting is that– coming back to your comment about an immature science with an immature taxonomy and vocabulary–that's why we've coined the term MLSecOps of which we think things like governance reporting, security, supply chain vulnerability, auditability, all those things are important from an MLSecOps perspective. Hence the name of the podcast.
And there are a bunch of key technical components that we think go into AI risk management á la MLSecOps. How do you think about the key technical components of AI risk management?
Patrick Hall 7:21
That’s a great question. What I like about your sort of framing is those things you said, monitoring, governance, reporting, those make sense. Those are things that organizations are likely already doing– larger organizations, more mature organizations are already doing to a certain extent. So I really like that framing, and honestly think it's more constructive and more productive.
I've been lucky enough to be involved, and it's been a real honor to be involved in the NIST AI risk management framework. And they lay out seven characteristics of trustworthiness. I'm not going to list them now because I'll do the thing where I get to six and can't remember the seventh one, but I would direct people to check out the seven trustworthiness characteristics that NIST has put forward.
And just since we're on this topic of vocabulary, a very important piece of research that went into the AI RMF AI risk management framework is a glossary. And so I worked with NIST and other co authors to put together about a 500 term glossary for Trustworthy AI or Responsible AI or Ethical AI terms, whatever we want to call it. So, minor progress there.
Charlie McCarthy 8:37
Awesome. So, Patrick, beyond the technical realm, let's switch over to culture. If we start thinking about culture items, capabilities maybe, that organizations need to build in order to have a more responsible AI implementation, what are some things that come to mind for you there?
Patrick Hall 8:46
Yeah, that's a very good question and point. So, I'm a big believer that culture reigns over strategy. And I think it was a fine in all the NIST research that an organizational culture that acknowledges risk is likely the most important thing you can do and have to manage risk.
I'm also a realist when it comes to culture. I don't think there's some kind of magical CEO tech bro dude out there who can just instill culture in an army of people. And so really, in the commercial world, culture comes from policies and procedures. Culture comes from incentives like pay and promotion. And culture comes from the top down. And instead of being sort of an inspiring CEO-type person, really it comes from the board risk committee.
So, if your board of directors doesn't have a risk committee, you probably don't really have a great risk culture. And of course, that's much more difficult for smaller organizations. I should carve out that these comments are really aimed at large organizations. And so, being a realist about culture, I would just say it's top down from the board and senior executives. There should be an executive in charge of risk. There should be policies and procedures about risk management in terms of technology and people should be incentivized to take on risk management tasks through pay and promotion.
Again, maybe not the most exciting answer, but I think that's how it actually works, at least in big technology organizations.
D Dehghanpisheh 10:12
And probably large organizations just collectively, right? Most public companies have a risk and compliance function. And it feels to me like on one hand there's kind of risk and compliance, but underneath that risk and compliance entity is the function of governance, right?
So, if you think about governance in that nested element and you think about governance of AI applications and machine learning systems in terms of mitigating risk, with the introduction of an AI application which is powered by an ML system, what are the aspects of governing those machine learning models and those machine learning systems as you see it? And why does that matter?
Patrick Hall 10:54
I’m a big proponent of governance of people. Now, of course, data and technology systems also need governance. But forgive me for not believing the paper that says this is the beginning of Artificial General Intelligence. Like, we're still in the days where if a computer misbehaves, you just go unplug it. And so, governance should be mostly about people. And there's many different ways to do this.
One that's likely not realistic for most companies. But I like to point it out because I think it is very effective. In large US Banks there's what's known as a Chief Model Risk Officer. And this is actually recommended by the Federal Reserve and other banking regulators. And so, this is a single person, a single throat to choke when things go wrong. And it's also important to say that this person is rewarded when things go well and that this person, in contrast to many directors of Responsible AI out there, this person has a large budget, very high stature in the organization, lots of staff. They have the ability to do things.
And so I would point out that if you can put one person in charge who gets in trouble when things go wrong and gets rewarded when things go right, that's a very, very serious sort of governance mechanism. Now, most companies will have to–because they don't have the regulatory mandate to do this–they'll have to come up with sort of software structures that involve committees and that involve sort of regular audits and things like this. And not to disparage those, because I think they're very important, and that's most of what companies can do. I just like to throw out this idea that I think is very effective of the Chief Model Risk Officer, which is a real thing in the largest banks in the US.
D Dehghanpisheh 12:38
Well, we’ve seen that with roles like that of Agus at Wells Fargo, who we both know. Agus is Executive Vice President, Head of Corporate Model Risk at Wells Fargo. And there are others in finance where this role of managing “model and AI risk” is getting increasingly important. Patrick, from your experience, what is that role in terms of guiding and determining everything from model selection to monitoring various feedback loops, et cetera? Talk to us a little bit about that.
Patrick Hall 13:06
Well, whoever it is, ideally it's a Chief Model Risk Officer, maybe it's an AI Risk Committee, maybe it's some other more standard risk function. They need technical people reporting to them. And what really is important, and again I see a lot of technology organizations struggle with this, is that the people on the testing and validation side have equal stature and equal pay with developers.
And it's really important that even if you can't do that, even if you can't pull off that trick where the testers are at the same level of organizational stature as the developers, you need very qualified people on the risk and validation side who can engage in what's known as effective challenge. Who can say, why did you pick this model? Why are you only monitoring this six months? Last time we had a model like this, it blew up after three months. Why did you use this algorithm that you just made up instead of this very similar algorithm from this big textbook that everybody likes?
So, ideally, these people operate with the same stature as the developers, but you need the testers and validators to have the same kind of technical background as the developers so that they can essentially ask hard questions. And that promotes effective challenge and critical thinking and common sense. A lot of these technology problems are human problems, as you well know.
D Dehghanpisheh 14:40
Yeah. So then, I would assume that to get those effective challenge muscles built inside of any organization, you kind of need two things. One, you need transparency and visibility. And the other is that you need kind of like a record, if you will, that allows everybody to frame the differences, almost like a git diff for ML. Do you think that's a reasonable way to think about that? And if so, it sounds like there needs to be a role for a machine learning dynamic bill of materials. Is that a fair assumption and connection in my brain here?
Patrick Hall 15:10
Well, I would say that documentation - and what you're talking about essentially sounds like documentation - documentation is known to play an essential role here. And you brought up Agus, Agus Sudjianto. I was on a panel with him at NIST, and during that panel he said that his bank had submitted 155,000 pages of model documentation to their regulators in one year.
And so, while that's not realistic for most companies, I think it just goes to show that documentation, whether it's some kind of specific diff, whether it's these long documents that the bankers write about their predictive models, whatever it is; having effective information that enables the building of these effective challenge muscles is really crucial. And I think any documentation is better than no documentation, and your idea sounds just fine to me.
D Dehghanpisheh 16:02
Yeah. You said it may not be commonplace for most enterprises to have that level of documentation that was referenced from that team that submitted it to their regulators. But is that commonplace, say, with large financial institutions or healthcare companies; entities that are very large and rely on ML every day, but have a massive regulatory environment that they have to respond to - is that a more commonplace thing in those regulated industries?
Patrick Hall 16:30
Yeah. And so, just to give audience members an idea–and I'm not necessarily advocating that all of this is good and helpful, okay? There's drawbacks to everything that we're talking about. But I think doing some of this is a lot better than doing none of this.
For what would be deemed a high risk model inside a large financial institution, just the empty Word document that you would be expected to fill out as you trained and documented your system – just the empty blank template – would be 50 pages long and would cover everything from your name and contact information, which I really like. I really like that part. And we've actually had very serious pushback from clients at BNH over this. Developers not wanting to put their name and contact information on their models, which I always find–
D Dehghanpisheh 17:19
Almost like a Sarbanes-Oxley of ML.
Patrick Hall 17:22
Yeah. And so anything from just who made this, to very extensive information about data limitations and assumptions expected, a very long bibliography in terms of your methodology. You would go from this 50 page blank template to a 150-300 page long document that covers every little detail about your model.
Charlie McCarthy 17:44
So, it sounds like some of these organizations probably are facing challenges, and there are some hurdles that they need to jump along the way to implementing responsible AI practices. Things like what you just mentioned, developers being hesitant to put name and contact information on things, the amount of documentation that's needed.
What other challenges do enterprises face when they're trying to implement this? Like, you mentioned earlier in the episode some of these larger companies laying off entire Responsible AI teams. Is there an organizational challenge that causes something like that? Or what are your thoughts there?
Patrick Hall 18:29
I think the general problem–and let's not even call it a problem. I think it's important not to call it a problem. The reality is there are market pressures to go fast and break things with machine learning and AI systems. Right? Unless there is a regulatory mandate not to do so, like you might find in large financial institutions, you might find in some sections of insurance and aviation and housing and employment. Unless there's real regulatory oversight, the market pressures to just get a product out there take over. Right?
And I think unless we're realistic about that, then we're not going to make progress. Right? And so, getting back to the idea of a law firm versus a consulting firm, what was really appealing to me is you have these very real market pressures that especially public companies are just going to have to work with. So how do you start with risk management or responsible AI?
And I think where people go wrong is not aligning it to the basic laws, basic product liability. [e.g.] You can't release dangerous products. You can't deceive people. You can't be predatory, and then sort of known embarrassing and costly failures. So, I think these high minded ideas around Responsible AI, Ethical AI, whatever we want to call it, fade away pretty quickly in the face of real commercial market pressures.
And in the face of real commercial market pressures my experience with what works is basing your programs off the basic legal obligations and the basic notion of we want to avoid incidents that embarrass us and cost us money. And without a regulatory mandate, that's about all you can do honestly. And for better or for worse that's the reality.
Charlie McCarthy 20:13
You just kind of touched on some risks that organizations might face by not implementing some of these practices. Aside from reputational damage or financial loss, are there other risks to enterprises you can think of?
Patrick Hall 20:18
Yeah. Lawsuits fly around like crazy. And the FTC has, it's an interesting term, disgorged three algorithms in three years. And I think more now, I kind of stopped keeping track. But the Federal Trade Commission has made up essentially a new enforcement action to go after the most flagrant offenders when it comes to machine learning. And again, not a lawyer, self-trained policy person, but what the FTC looks for is known as UDAP: Unfair, Deceptive and Predatory.
So, you can be unfair and deceptive and predatory as a car dealer. You can be unfair, deceptive, and predatory as a maker of AI tools. Right? And so, those are some of the basic gotchas to watch out for. Now, I want to be clear that I think 80% of you know–this is an 80-20 thing. 80% of companies out there working with AI machine learning technologies are not looking to be unfair, deceptive or predatory but it can happen by accident. It can happen by accident, and then, of course, there are the bad actors in the market.
D Dehghanpisheh 21:26
So then, it's almost like thinking about the fact that if you're going to be deploying AI systems in some meaningful capacity, it's kind of like a data breach or a cybersecurity breach. At some point you're going to be hacked. And so, it almost feels like at some point on a long enough time horizon there's going to be an AI or ML incident that hits you. And the mileage may vary in terms of the severity.
So if it's not an if but when, how do organizations prepare for an inevitable security incident of some kind? And then how do they develop a successful ML security Incident Response Plan? I mean, going back to the three disgorged algorithms that you mentioned before, how does somebody think about their incident response management element in the age of AI?
Patrick Hall 22:15
I mean, not too differently from the way you think about standard security incidents. And I think that's where we really go wrong oftentimes just as a group of practitioners, sort of imbuing our technology with magical powers that it absolutely does not have. Data scientists are not magicians, and our models are not magic. Really they're just fancy IT systems. And if we just manage them and govern them like the rest of our IT systems, we would be a lot better off.
Now, of course, there are special carve outs, special risk, special things that can go wrong with AI and machine learning systems. But again, I'm a big proponent of the 80-20 rule. It kills me when I walk into a client or a corporation and ask if they have an AI incident response plan for this AI system that they spent $10 million on and is part of their mission critical business activities. The answer is always no, but the database three feet away from it in the data center has an incredibly detailed and rehearsed incident response plan.
We just have to get over this magical thinking with AI, right? It's not magic. It can fail. It can harm people, it can be abused by people. And you need to have plans there to deal with it, and they're not that different from your existing security plans. And I just wish that we would get over this kind of magical thinking. Right? They're just IT systems. Just manage and govern them like other IT systems, and you'd be 80% of the way there.
D Dehghanpisheh 23:40
Yeah, okay, so that gets you 80% of the way there, but I'm assuming it's that last 20% that's far more critical. Otherwise, people wouldn't be so up in arms about the potential AI risk or ML risk. Right? I mean, nobody else is going around saying, well, this app could really destroy things, or this thing could really upend X, Y, or Z. It seems like, sure, there might be that 80%, but even if I'm doing that 80%, this remaining 20% is where I'm probably most vulnerable because I didn't go far enough.
Patrick Hall 24:09
I think you can definitely be called out in that remaining 20%. I don't want to do anything to discourage people from just governing AI systems like they were regular old IT systems, but I agree that you can definitely be called out by that 20%. And so, some of these machine learning attacks, like recently there was released an availability attack on machine learning systems which I hadn't really seen before. And these were called sponge example attacks.
So, some smart researchers had devised some kind of inputs to neural networks that either slow down the inference to an unmanageable point or make them use an unreasonable amount of energy to make an inference. And so that's an example of a special machine learning risk. But at the same time, if you were monitoring your CPU usage, and your memory usage, and your power usage on these systems, you would know that something was going wrong.
So again, I think it's this kind of 80-20 rule where, just do the basics. That's a lot better than not doing the basics, I guess, and a lot of people aren't doing the basics, in my opinion.
D Dehghanpisheh 25:13
So within that 80-20 rule, right, you've got new players that need to kind of augment the responsibilities within the 80 and maybe take over responsibility in the 20. You've got data scientists, you've got security professionals, and business leaders, even themselves, who are playing a role in implementing ML systems. And thus they need to be involved in the ML security incident response.
What roles do kind of the unique ML practitioners, whether they're data scientists, machine learning engineers, or ML business leaders; what role do they need to play within that existing 80% and then the new 20%?
Patrick Hall 25:52
Sure. So I think they need to get out of the way and let some basic governance and management happen. That's one part of it. And then where they really need to use their expertise is in helping legal colleagues, cyber information security colleagues, data privacy colleagues, helping them understand their risk.
A lot of what BNH does is get called in by data privacy attorneys, called in by fair lending attorneys, called in by general product counsel to understand the specific risk of AI and ML systems because their own internal people aren't telling them. And this gets back to people not wanting to put their name and email address on their code.
We're talking about a group of people, generally speaking, that are paid more on average than general practice physicians and they want no accountability. They want no responsibility and no accountability.
And so maybe I was kind of speaking negatively about commercial market pressures earlier. Commercial market pressures aren't going to allow for that for very much longer either, right? If you're making more money than a physician, you need to put your name on your work. And you need to help your legal colleagues understand what the risks they're dealing with are. And your security colleagues. And your data privacy colleagues.
So we've got some serious cultural problems in machine learning and AI. Maybe we've kind of beat around the bush on that, but we can jump into it if you want.
D Dehghanpisheh 27:12
Sounds like we just did.
Patrick Hall 27:14
Yeah.
Charlie McCarthy 27:15
I'd like to jump over, Patrick, and switch gears a bit to a trending topic that is ripe for incidents and risk; large language models. We're probably all tired of talking about them, but can't really avoid it at this point.
So LLMs, what unique challenges do they pose for Responsible AI, particularly in terms of things like data privacy, bias management, and other incidents?
Patrick Hall 27:44
I'm not even sure where to get started here, but I think one thing to keep in mind is everybody is focused on ChatGPT. That's fine. I'm a ChatGPT pro user, I find it occasionally helpful. I think when it comes to ChatGPT, what makes it special and potentially different than other chat bots that came before it or may also be out there, is the amount of human labor that went into content moderation.
And so, ChatGPT is a little bit different because it seems that some of the bias was managed there, which has been a huge mistake in previous chat bots. Previous generations of chat bots, or even chat bots that were released right before and right after ChatGPT were all teed up to go on racist, xenophobic, sexist, crazy sprees because of the data they were trained on and how risk was not managed properly. All this to say that I think ChatGPT has done an okay job with bias management, and much more so than previous generations.
I also want to call out that it’s almost all due to human content moderation, not anything special about the AI or the language model. But even with the bias managed–if we're willing to call it that, which I'm sure people would disagree with me on, and perhaps rightly so. But let's just say for the sake of discussion that we've done a little bit better with bias management–there's still very serious issues with both data privacy and intellectual property infringements. What happens to my data when I type into ChatGPT? And then what happens when I accidentally use someone else's proprietary data because somehow, magically, it's now appearing in the outputs of ChatGPT.
So just to be clear, there have been public accusations from both Amazon and Samsung that they can regenerate proprietary information from the outputs of ChatGPT. So you should be very careful with the information you put in and very careful with the information you get out.
There's also the issue of - I hate this word - hallucinations. I don't know, why don't we just call it errors? The things are wrong all the time, right? And I'll give you an example. And neural networks have a long history of just hand-wavy vocabulary and using it for gatekeeping and that's a whole different topic. But, yeah, hallucinations. They're just wrong all the time.
So to give an example of how sort of difficult to spot it can be, I was using it to help me draft a recommendation letter for a student late one night, I was very tired. And I just happened to notice that ChatGPT generated text said the student was commended for X, Y, and Z. And it just didn't sound right. So, I went back and looked at the resume, and they weren't commended for X, Y, and Z. They had just done X, Y, and Z. So, you have to proofread everything it says for any kind of high risk application.
And then there's also this issue of what NIST calls automation complacency. It's really, really important to understand these systems are trained to generate the most likely text given the input text, right? That's what they do. That's their intelligence, if you want to call it that, which we could argue about whether you should call it that. These systems are designed to generate content. That is a very, very different design than for a system which is designed to support decisions or, take a deep breath, automatically make decisions. These systems have no valid mechanism to support decision making. You have to be really careful that they don't accidentally make decisions for you, say, sending an email or something like that.
And those are sort of the mundane risks. Data privacy, intellectual property, errors, and automation complacency. Then we can get into all the fun stuff around mis- and disinformation, deep fakes. Those are the scary risks. And I want to be really clear that I think the talk of emergent capabilities and the AI systems learning to accelerate themselves and not turn themselves off, I think that is a complete sort of smokescreen put out there for bad faith reasons to confuse policymakers and confuse the public just to be as direct as possible about it.
So there are catastrophic existential X-risk with these systems, but they're about disinformation. They're not about the system book turning into HAL. I find that discussion just endlessly annoying, and I'm sure you can tell by the tone of my voice.
D Dehghanpisheh 32:23
So you've mentioned the NIST framework a couple of times as a practical guide to help people implement that, and your book talks a little bit about that. How do you recommend organizations get started using the NIST framework to improve their AI security posture and their AI responsibility posture?
Patrick Hall 32:44
Well, I hate to break everybody's heart, but you're going to have to read. You’re going to have to read some government documents.
D Dehghanpisheh 32:53
Those are always the best authors.
Patrick Hall 32:57
Yeah. So, there's the core AI RMF (Risk Management Framework), which is a PDF, NIST AI 100-1. That's sort of the core document, I would say, for executives and policymakers and policy people inside of organizations. For practitioners, you want to look at what's called the Playbook, the NIST AI RMF Playbook, which is a set of documents and websites that gets into more implementation level details.
And then there's a ton of really great–take it with a grain of salt, because my name is on some of it–but I think it's some of the best AI research in terms of Trustworthy AI, or Responsible, or whatever you want to call it, that supports the AI RMF. There's the vocabulary paper, there's multiple papers on transparency, the bias management paper I think is incredible. And it has an extremely different tone than what's coming out of commercial AI and ML labs. So, if nothing else, if you'd like a fresh perspective, go check out some of this work from NIST. There's a lot of parts of that framework, but the Playbook is probably what we want to talk about as practitioners.
D Dehghanpisheh 34:02
If you had to summarize the difference in tone between the assets that you were just referencing and the tonality from some of the big AI vendors and language model and foundational model creators. What's the difference in the tone?
Patrick Hall 34:20
Science. Science is the difference in the tone. In the work with NIST, in interacting with the National Academy of Science, interacting with the White House or Science Policy Office. They have very serious questions about the fundamental scientific credibility of a lot of what data scientists do with machine learning and AI, right? Black box or unexplainable systems where you pick the best outcome by trial and error using data that's very similar to the data that it was sampled on, where it's not reproducible and you don't tell anyone how it works. That's just not science.
That's just not science. It might be engineering. In the best case, it's engineering. Some of these might be incredible engineering feeds, and some of them might turn out to be great scientific feeds. We just won't know because there's no transparency, verifiability or reproducibility yet. So, I would say the main tone is: show us the causal scientific evidence that these claims you're making actually work, that these claims are real, that these systems do what they do. And have that said by a real scientific body that's not set up to make money by claiming that it works. And it's a big difference in tone. It's a very big difference in tone.
D Dehghanpisheh 35:37
Springboarding off of that, if you will…
We've talked a lot about the technical practitioners and the technology underpinnings and the various system elements. Now, as we close out, there are those who listen to this podcast frequently–thank you for your contributions–and who read the transcripts often and ask us a lot of questions. And they come from areas of the company that include risk management, compliance, and general counsel positions increasingly.
What should they be thinking about? What should they be doing? And then I want to have you close out with how can BNH.AI help them?
Patrick Hall 36:14
Very kind of you. Again, I want to get back to this theme of AI isn't magic. If you're a product counsel and you've dealt with IT systems before, you can do good work on AI systems. If you're a data privacy attorney and you've worked with any algorithmic decision making, you're okay to start working on AI systems.
So, I think the key there for those people is, throw the magic out the window. Throw the hype out the window. This is a freaking computer like everything else you've worked with. Now, as we've said before, there are these specialized AI risks, and really interesting cultural characteristics of data scientists.
And it's not always the data scientist. It can be their management. It can be the business side. There's some interesting cultures around AI, and so I think where BNH really shines, if I can say so, is (1) by helping people take their AI risk management to the next level and really address those specific AI risks, and then (2) helping people navigate this just weird culture that grows up in organizations around this technology.
A lot of what we do, I think, is just cultural translation between risk, compliance, legal, and technology. So, getting into the specifics, building a really bulletproof AI risk management framework, and then helping you understand the culture of the different people and tribes and groups you're working with, I think that's what BNH does.
D Dehghanpisheh 37:46
Our guest today was Patrick Hall, senior data scientist and one of the founders of BNH.AI.
I want to thank you for coming on today.
Patrick Hall 38:01
My pleasure.
D Dehghanpisheh 38:02
If you're interested in learning more, continue to watch the MLSecOps.com site for updates and future guests. And last but not least, this is brought to you by Protect AI, who is focused on that last 20%, and that critical 20%. So reach out. Thanks for coming on, Patrick.
Charlie McCarthy 38:18
Thanks, Patrick.
Patrick Hall 38:19
You’re very welcome.
D Dehghanpisheh 38:20
See you.
Closing 38:21
Thanks for listening to The MLSecOps Podcast brought to you by Protect AI. Be sure to subscribe to get the latest episodes and visit MLSecOps.com to join the conversation, ask questions, or suggest future topics. We're excited to bring you more in-depth MLSecOps discussions. Until next time, thanks for joining.
Additional tools and resources to check out:
Protect AI’s ML Security-Focused Open Source Tools
LLM Guard - The Security Toolkit for LLM Interactions
Huntr - The World's First AI/Machine Learning Bug Bounty Platform
Thanks for listening! Find more episodes and transcripts at https://mlsecops.com/podcast.